Environments
MALib implements a unified environment interface to satisfy both turn-based and simultaneous-based environments. MALib works with different environments, including simple Markov Decision Process environments, OpenAI-gym, OpenSpiel, and other user-defined environments under MALib’s environment API. We first introduce the available environments supported by MALib and then give an example of how to customize your environments.
Available Environments
This section introduce the environments have been integrated in MALib.
Simple Markov Decision Process
mdp is a simple and easy-to-specify environment for standard Markov Decision Process. Users can create an instance as follows:
from malib.rollout.envs.mdp import MDPEnvironment, env_desc_gen
env = MDPEnvironment(env_id="one_round_mdp")
# or get environment description with `env_desc_gen`
env_desc = env_desc_gen(env_id="one_round_mdp")
# return an environment description as a dict:
# {
# "creator": MDPEnvironment,
# "possible_agents": env.possible_agents,
# "action_spaces": env.action_spaces,
# "observation_spaces": env.observation_spaces,
# "config": {'env_id': env_id},
# }
Note
In MALib, this environment is used as a minimal testbed for verification of our algorithms’ implementation. Users can use it for rapid algorithm validation.
The available scenarios including:
one_round_dmdp
: one-round deterministic MDPtwo_round_dmdp
: two-round deterministic MDPone_round_nmdp
: one-round stochastic MDPtwo_round_nmdp
: two-round stochastic MDPmulti_round_nmdp
: multi-round stochastic MDP
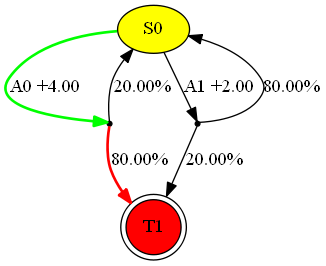
Illustration of a Multi-round stochastic MDP
If you want to customize a MDP, you can follow the guides in the original repository.
OpenAI-Gym
Gym is an open-source Python library for developing and comparing reinforcement learning algorithms by providing a standard API to communicate between learning algorithms and environments and a standard set of environments compliant with that API. Since its release, Gym’s API has become the field standard for doing this.
from malib.rollout.envs.gym import GymEnv, env_desc_gen
env = GymEnv(env_id="CartPole-v1", scenario_configs={})
env_desc = env_desc_gen(env_id="CartPole-v1", scenarios_configs={})
# return an environment description as a dict:
# {
# "creator": GymEnv,
# "possible_agents": env.possible_agents,
# "action_spaces": env.action_spaces,
# "observation_spaces": env.observation_spaces,
# "config": config,
# }
DeepMind OpenSpiel
OpenSpiel is a collection of environments and algorithms for research in general reinforcement learning and search/planning in games. OpenSpiel supports n-player (single- and multi- agent) zero-sum, cooperative and general-sum, one-shot and sequential, strictly turn-taking and simultaneous-move, perfect and imperfect information games, as well as traditional multiagent environments such as (partially- and fully- observable) grid worlds and social dilemmas. OpenSpiel also includes tools to analyze learning dynamics and other common evaluation metrics. Games are represented as procedural extensive-form games, with some natural extensions.
from malib.rollout.envs.open_spiel import OpenSpielEnv, env_desc_gen
env = OpenSpielEnv(env_id="goofspiel")
env_desc = env_des_gen(env_id="goofspiel")
# return an environment description as a dict:
# {
# "creator": OpenSpielEnv,
# "possible_agents": env.possible_agents,
# "action_spaces": env.action_spaces,
# "observation_spaces": env.observation_spaces,
# "config": config,
# }
PettingZoo
PettingZoo is a Python library for conducting research in multi-agent reinforcement learning, akin to a multi-agent Gym environment <https://github.com/Farama-Foundation/Gymnasium>. It integrates many popular multi-agent environments, also modified multi-agent Atari games.
Available Environments
Atari: Multi-player Atari 2600 games (cooperative, competitive and mixed sum)
Butterfly: Cooperative graphical games developed by us, requiring a high degree of coordination
Classic: Classical games including card games, board games, etc.
MPE: A set of simple nongraphical communication tasks, originally from https://github.com/openai/multiagent-particle-envs
SISL: 3 cooperative environments, originally from https://github.com/sisl/MADRL
Note
For the use of multi-agent Atari in PettingZoo, you should run `AutoROM
to install rom, and pettiongzoo[classic]
to support Classic games; pettingzoo[sisl]
to support SISL environments.
There is a file named scenarios_configs_re.py
under the package of malib.rollout.envs.pettingzoo
which offers a default dictionary of supported scenarios and configurations. Users can create a pettingzoo sub enviornment by giving an environment id in the form as: {domain_id}.{scenario_id}. The domain_id
could be one of the above listed five environment ids, and the scenario_id
can be found in the full list of them from the documentation of pettingzoo.
from malib.rollout.envs.pettingzoo.scenario_configs_ref import SCENARIO_CONFIGS
for env_id, scenario_configs in SCENARIO_CONFIGS.items():
env = PettingZooEnv(env_id=env_id, scenario_configs=scenario_configs)
action_spaces = env.action_spaces
_, observations = env.reset()
done = False
while not done:
actions = {k: action_spaces[k].sample() for k in observations.keys()}
_, observations, rewards, dones, infos = env.step(actions)
done = dones["__all__"]
As pettingzoo supports two simulation modes, i.e., AECEnv
and ParallelEnv
, users can switch it with specifying parallel_simulate
in scenario_configs
. True
for ParallelEnv
, and False
for AECEnv
.
SMAC: StarCraftII
coming soon …
Google Research Football
coming soon …
Environment Customiztion
MALib defines a specific class of Environment
which is similar to gym.Env
with some modifications to support multi-agent scenarios.
Customization
Interaction interfaces, e.g., step
and reset
, take a dictionary as input/output type in the form of <AgentID, content>
pairs to inform MALib of different agents’ states and actions and rewards, etc. To imeplement a customized environment, some interfaces you must implement including
Environment.possible_agents
: a property, returns a list of enviornment agent ids.Environment.observation_spaces
: a property, returns a dict of agent observation spaces.Environment.action_spaces
: a property, returns a dict of agent action spaces.Environment.time_step
: accept a dict of agent actions, main stepping logic function, you should implement the main loop here, then theEnvironment.step
function will analyze its return and record time stepping information as follows:def step( self, actions: Dict[AgentID, Any] ) -> Tuple[ Dict[AgentID, Any], Dict[AgentID, Any], Dict[AgentID, float], Dict[AgentID, bool], Any, ]: """Return a 5-tuple as (state, observation, reward, done, info). Each item is a dict maps from agent id to entity. Note: If state return of this environment is not activated, the return state would be None. Args: actions (Dict[AgentID, Any]): A dict of agent actions. Returns: Tuple[ Dict[AgentID, Any], Dict[AgentID, Any], Dict[AgentID, float], Dict[AgentID, bool], Any]: A tuple follows the order as (state, observation, reward, done, info). """ self.cnt += 1 rets = list(self.time_step(actions)) rets[3]["__all__"] = self.env_done_check(rets[3]) if rets[3]["__all__"]: rets[3] = {k: True for k in rets[3].keys()} rets = tuple(rets) self.record_episode_info_step(*rets) # state, obs, reward, done, info. return rets
MALib also supports Wrapper functionality and provides a GroupWrapper to map agent id to some group id.
Vectorization
MALib supports interacting with multiple environments in parallel with the implementation of auto-vectorized environment interface implemented in ‘malib.rollout.env.vector_env’.
For users who want to use parallel rollout, he/she needs to modify certain contents in rollout_config.
rollout_config = {
"fragment_length": 2000, # every thread
"max_step": 200,
"num_eval_episodes": 10,
"num_threads": 2,
"num_env_per_thread": 10,
"num_eval_threads": 1,
"use_subproc_env": False,
"batch_mode": "time_step",
"postprocessor_types": ["defaults"],
# every # rollout epoch run evaluation.
"eval_interval": 1,
"inference_server": "ray", # three kinds of inference server: `local`, `pipe` and `ray`
}